Recruiters today often find themselves bogged down by administrative tasks, spending 20% to 40% of their time on activities that don't directly drive hiring success. This issue is more than just a minor inconvenience—it’s a significant drag on productivity.
Fortunately, advances in artificial intelligence, machine learning, and natural language processing are offering solutions to this age-old problem.
Technology now has the power to automate or even eliminate routine tasks like drafting job descriptions, scheduling interviews, and taking notes. By reducing the administrative burden, these tools can help recruiters focus on the high-impact activities that truly drive recruitment success.
To understand how this is possible, it’s crucial to understand the two main types of data that play a role: structured and unstructured data, and the key differences between them. So that’s what we’re going to cover in this article.
Types of data in recruitment: Unstructured vs structured data
First things first, what are structured and unstructured data?
.jpeg)
Structured data
Also known as quantitative data, structured data is organized according to a standardized format or schema, which makes it straightforward to enter, analyze, and query using basic algorithms.
This type of data is typically stored in pairs of data points and values, arranged in databases with rows and columns. This organization facilitates easy processing, filtering, searchability, and data analysis.
For example, a customer database with fields such as name, address, phone number, and credit card number represents structured data. The consistent format or data structure allows both humans and machines to efficiently process and understand it.
Common tools for managing structured data include relational database management systems like MySQL and PostgreSQL, spreadsheets such as Microsoft Excel, and CSV files.
These tools work with a predefined data model and use specific query languages like SQL (Structured Query Language) to manipulate and retrieve data efficiently. SQL allows users to perform operations such as querying, updating, and managing data within these structured environments.
In recruitment, examples of structured data include:
- Candidate information: Details such as names, contact information, job titles, and work history, all organized in a consistent format for easy retrieval and management.
- Recruitment metrics: Data on key performance indicators like time-to-hire, cost-per-hire, and source of hire. This information is typically tracked and organized in a way that allows for straightforward analysis using analytics tools.
Despite some limitations, structured data is crucial for efficient recruitment. It supports a range of use cases and functions, from quick and precise candidate filtering to progressing job seekers through the recruitment funnel by tracking their status at each stage and facilitating smooth transitions between stages.
Additionally, structured datasets help generate detailed analytics and reports on key metrics like time-to-hire, cost-per-hire, and source of hire, which streamline the hiring process and enhance decision-making.
Note: An important aspect of structured data is metadata, which provides additional context about the data itself.
Metadata describes the characteristics of data, such as its source, format, and relationships with other data points. For example, in an ATS, metadata might include information about when a candidate applied or how their data was sourced.
By understanding and leveraging metadata, you can enhance data management and improve the accuracy of your analyses and reporting.
Unstructured data
Unstructured data, also known as qualitative data, does not follow a standardized format or schema. This type of data can come in various forms and file formats such as text files, audio files, or video files, and often lacks the organization that makes structured data easy to analyze.
Because of its diverse and unorganized nature, unstructured data is typically stored in NoSQL databases like MongoDB, data warehouses or data lakes, which are designed to handle large volumes of raw, unstructured information. These data management tools allow for the preservation of unstructured data without imposing a rigid data format.
In the recruitment context, examples of unstructured data include:
- Resumes and cover letters: Although some resume templates come with predefined formats, these documents are often unstructured because they contain free-form text and vary widely in style and content. While templates can provide some consistency, the content within resumes and cover letters can be diverse and unstructured, requiring advanced parsing and analysis to extract relevant information.
- Interviews and intake calls: Data sources like interviews and intake calls, which are often recorded in various formats, need to be turned into structured data like meeting notes manually.
- Social media profiles: User profiles and social media posts from platforms like LinkedIn, Twitter, or Facebook, can provide insights but require additional processing to integrate with structured data.
While unstructured data can be more challenging to process, it offers rich, contextual insights that complement the information obtained from structured data.
By leveraging advanced data analytics, machine learning algorithms, and natural language processing tools, recruiters can extract valuable information from unstructured sources, enhancing their understanding of candidates.
Just as with structured data, metadata is crucial for managing unstructured data.
Metadata in this context can include information about the source of a resume or the context of a social media post. It helps in organizing and retrieving unstructured data more effectively, ensuring that valuable insights are not lost or overlooked.
Note: There is a third kind of data, called semi-structured data, that you should know about. This kind of data is not captured or formatted in a traditional, tabular view. In fact, it doesn’t have a fixed schema at all. JSON, XML, and emails are common examples of semi-structured data.
Why both types of data matter in recruitment
So, why should the differences between structured and unstructured data matter to you, as an in-house or agency recruiter?
Three main reasons:
- Firstly, because you can’t avoid having both types of data. In any recruitment process, you’ll inevitably encounter structured data like candidate databases and ATS information, and unstructured data such as resumes and interview notes.
- Secondly, because they provide complementary insights into candidates. Structured data offers clear, organized information that facilitates efficient data management and analysis, while unstructured data provides rich, contextual details that give a deeper understanding of a candidate’s fit and potential. Together, they enable a more comprehensive and nuanced evaluation of candidates.
- And thirdly, because feeding both types of data into AI tools enables recruitment teams to fully automate the hiring process from end-to-end. For example, integrating structured data from an ATS with unstructured data from resumes and chat interactions allows AI tools to seamlessly match candidates to job openings, automate interview scheduling, and even draft personalized communication. This integration not only speeds up the hiring process but also ensures a more comprehensive and accurate candidate evaluation, reducing manual effort and enhancing overall recruitment efficiency.
Now let’s look at the types of tools used for managing recruitment data.
Tools for managing recruitment data
There are various tools available for managing recruitment data within your company. Here’s a look at popular options for handling both structured and unstructured data.
Structured data tools
- Applicant tracking systems: ATSs are essential for managing and streamlining the recruitment process. They centralize candidate information, such as resumes and application details, into a single, searchable database. This organization simplifies tracking candidates through each stage of the hiring process, from initial application to final offer, and enhances team collaboration and productivity.
- Customer relationship management software: While CRM software is traditionally used for managing customer interactions, it can be adapted for recruitment to track and engage with candidates. CRMs help in maintaining a detailed record of candidate interactions, follow-ups, and communications. They offer valuable insights into candidate pipelines and can support relationship-building efforts throughout the recruitment lifecycle.
- Reporting solutions: Reporting tools provide insights into your recruitment efforts by tracking key metrics such as time-to-hire, cost-per-hire, and overall process efficiency. These solutions help you assess and improve the effectiveness of your hiring strategies.
Unstructured data tools
- AI recruitment tools: AI-powered platforms assist with various stages of the recruitment process, including posting job ads, sourcing candidates, and sending personalized communications. These tools automate many tasks, reducing the need for direct human involvement.
- AI assistants: AI assistants can handle repetitive tasks such as writing job descriptions, scheduling interviews, and transcribing meeting notes. By taking over these time-consuming activities, they allow you to focus on more strategic aspects of recruitment.
- Chatbots: For companies that can’t provide 24/7 service, chatbots offer a solution by engaging with potential candidates around the clock. They collect valuable information and facilitate interactions that can be integrated into the hiring process.
Integrations across the recruitment tech stack
Both categories of tools—structured and unstructured data solutions—come with their own set of advantages and challenges. In the day-to-day reality of a recruiter, you’ll find that both types of tools are necessary for a well-rounded recruitment strategy.
Structured data tools provide organization and efficiency, helping you manage and analyze candidate information systematically. On the other hand, unstructured data tools offer rich insights and automation, enhancing your ability to engage with candidates and streamline various tasks.
Integrating these tools across your recruitment tech stack ensures a seamless flow of information and processes.
For example, data collected by chatbots and AI assistants during the sourcing and screening phases of the recruitment process can be fed into your ATS to optimize the candidate information coming from resumes and social media profiles.
AI assistants can join recruiters during intake calls and interviews, taking notes and turning those notes into docs with specific formats, like meeting summaries, job descriptions, and candidate write-ups.
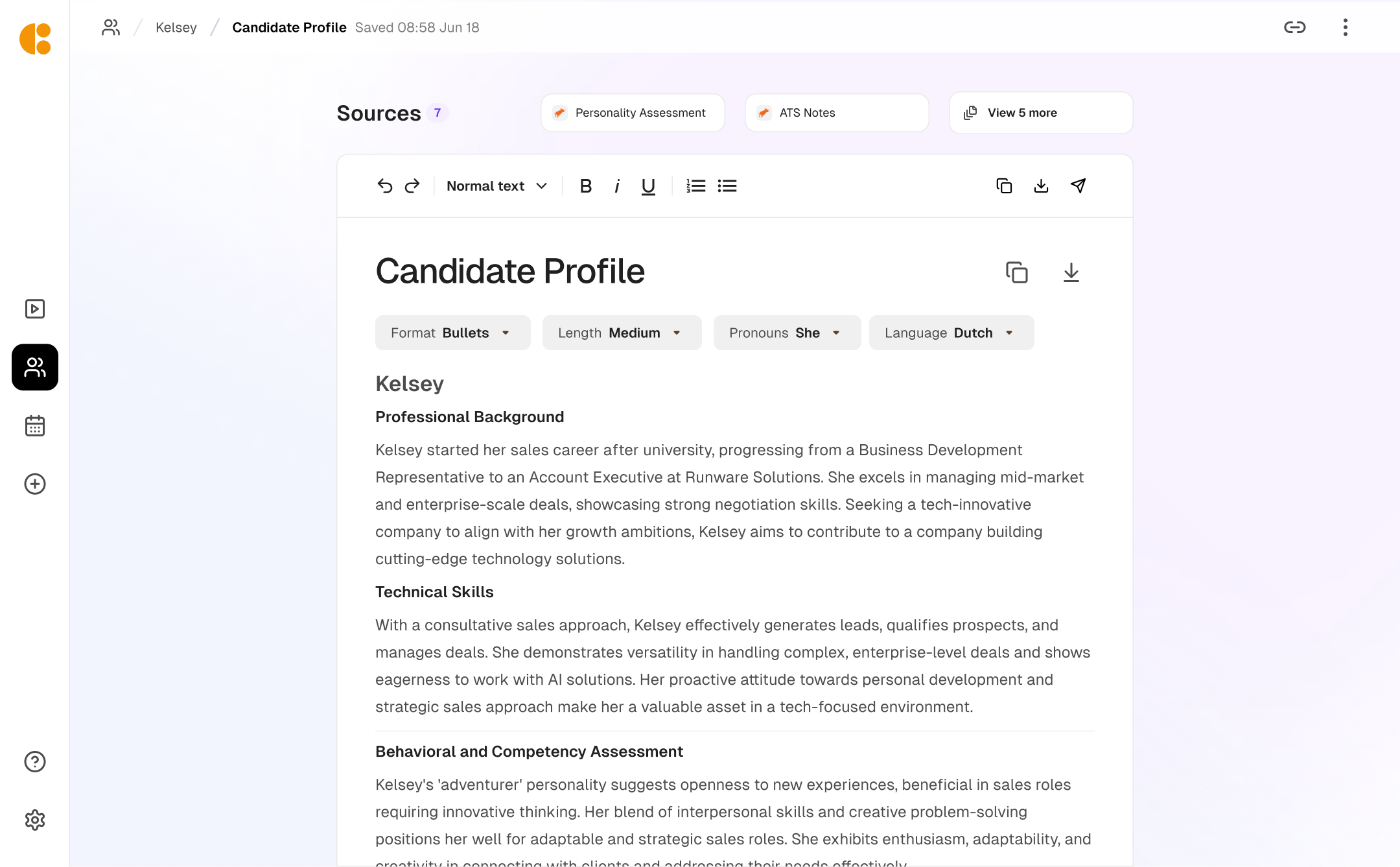
This not only reduces the time recruiters spent on admin tasks, but also minimizes the risk of errors and ensures data hygiene in the ATS.
By leveraging the strengths of both types of tools and ensuring they work together, you can create a more efficient, data-driven recruitment process that enhances your ability to attract, evaluate, and hire top talent.
Over to you
To recap, both types of data are crucial for the recruitment process, each offering unique benefits. Effective recruitment relies on understanding and leveraging both data types with appropriate management strategies and tools.
.jpeg)